(tldr; Why TeamCity is still a time and money saving tool that can also be used for Continuous Delivery)
Today Continous Integration is a widely adopted practice and Continuous Delivery is the new state of the art. Many companies are now using (or 'abusing'?) their existing CI infrastructure to build pipelines for Continuous Delivery.
It turns out that this exercise works very well with tools like Jenkins, Hudson or Teamcity. The concept of having 'Builds' that are triggered by 'Commits' and producing 'Artifacts' can be easily extended to create delivery pipelines. Add the ability to chain 'Builds' and extend the trigger mechanism to fire when all preceding builds have been successfully finished. A 'Build' can be a classic compile build, a complex acceptance test, or the deployment to machines. It is just some kind of 'Job'. The possibility of 'Scheduled' triggers transforms your CI system to be a generic automation system. The traceability of 'Jobs' with logs and build numbers, some basic metrics and a nice Web-GUI lured many sysadmins exposed to CI systems to use them for automating all kind of stuff.
That is the way
AutoScout went with
TeamCity during the last years and with good success. Today, AutoScout has more than 1000 active build configurations executed by 51 agents. Many of those 'builds' aren't actually building stuff, but are
- deploying apps to servers
- updating elasticsearch indices
- running database schema migrations
- orchestrating fleets of test agents
- and other crazy stuff you won't believe ;-)
We are not always happy with TeamCity. It tends to be a clicky-clicky tool producing
clickops, because it has a nice GUI but a hard to use REST-API. And if you use
Builds for orchestrating other machines to do the real stuff
(e.g. deployments) then you do not make good use of your agents resources. The agent is then blocked by idle waiting, wasting resources that cost real money. Over the years, TeamCity accumulated so many features that you need experience to do things the right way without shooting yourself in the foot.
A few months ago AutoScout decided to change its tech stack from DataCenter/Windows/.NET to Cloud/Linux/JVM. As this change is quite big and risky we have hired a lot of expertise from
ThoughtWorks, the company that is also a well known proponent of
Continuous Delivery. Their experts suggested to abandon TeamCity and give
GO a try because GO is open source and built with Continuous Delivery in mind.
Now, after some hands on experiences with GO, I believe that you shouldn't replace a working and mature CI System like TeamCity with GO. My main argument is that GO is just another CI-Tool with no fundamental difference to TeamCity, Jenkins or whatsoever. It shares the same disadvantages
(clicky-clicky, server-agent model) but lacks many productivity features that TeamCity provides in a mature and battle proven way. Indeed I miss so many things in GO that I liked in TeamCity that I start a rant right now ;-)
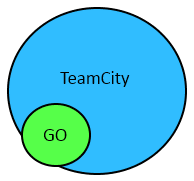 |
GO features are mostly a small subset of TeamCity features |
Displaying Logs
Investigating build logs is one very important use case whether you need to find the reason why a build fails or you want to know what a build makes slow. GO is a complete disappointed in this point. It shows you only a raw black&white dump of stdout and stderr mixed together. No indicators or any kind of help that could support you in analyzing. Maybe because only can start shell scripts. TeamCity, in contrast, knows what it executes (e.g. msbuild, rake, test runners, ...). It uses this knowledge to enhance logs with hints that makes them easy to analyze. First of all, errors are extracted and shown in a prominent place, so most often you don't need to go to the build log at all. If you drill down to the log, the log is presented in a hierarchical view, where blocks containing errors are expanded (and colored in red) while the rest of the log is collapsed. TeamCity also shows you for each line its ingestion time and the accumulated durations of tasks and subtasks. That makes it very easy to see where the build spent time. GO can't even display a timestamp, so if your build takes 20 minutes, you have no clue which task caused this delay. TeamCity just tells you which task took how long, so you know immediately the reason for the performance degradation.
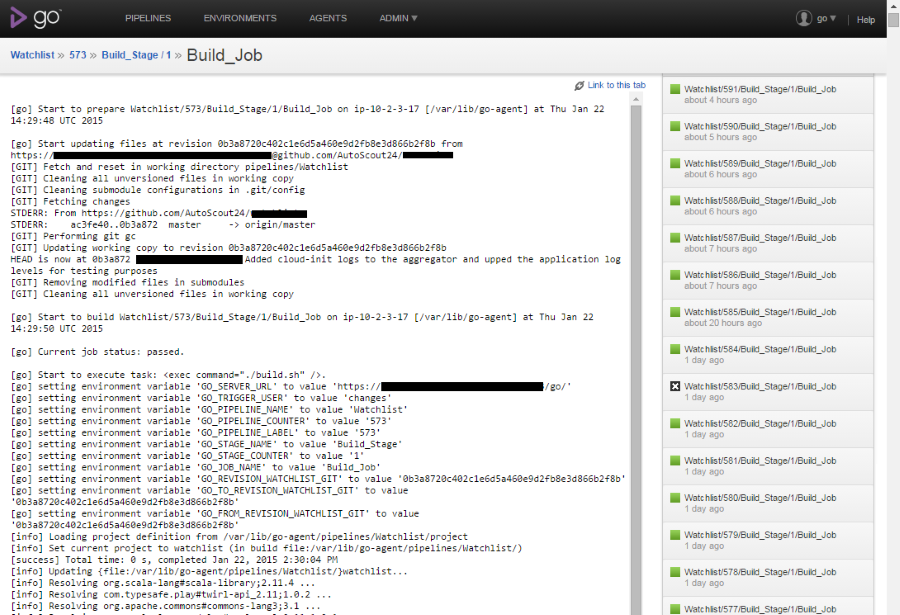 |
GO displaying a build log |
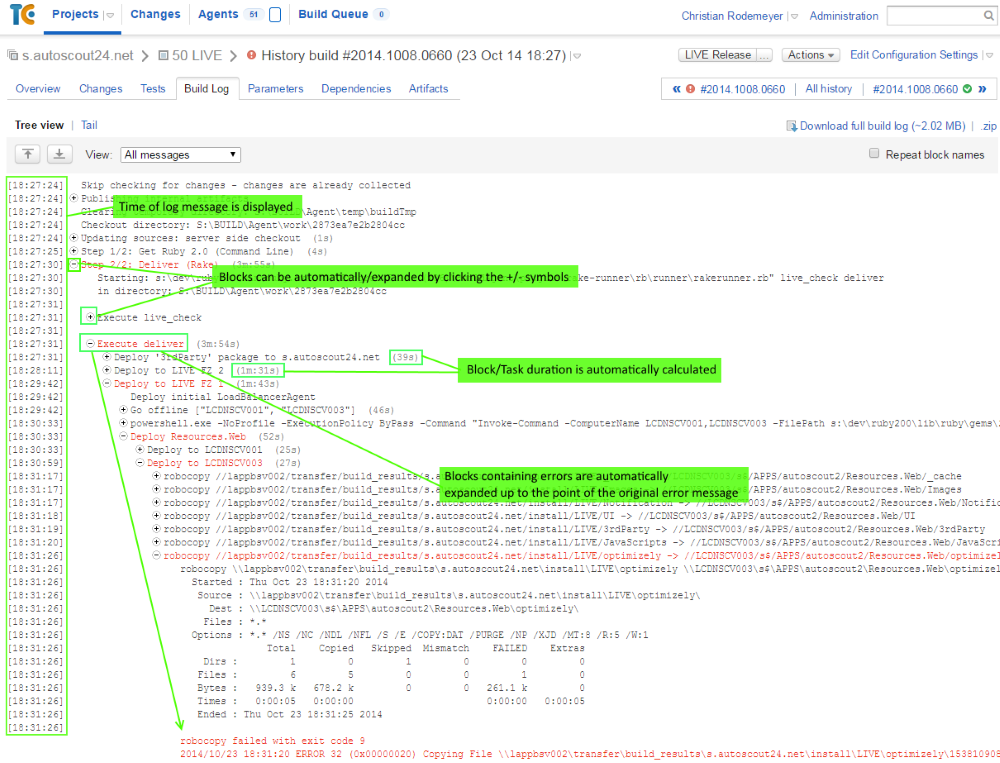 |
TeamCity displaying a build log |
Red Builds aka Failed Jobs
Red builds or failed jobs need to be investigated. In GO you can do silly things like wearing hats or hope that somebody takes care, in TeamCity you can start investigating a build and then the rest of the team knows that you are taking care.
Speaking or red builds, TeamCity has many ways to mark a build as failed even if the job returned a success, e.g.
- the number of tests suddenly dropped significantly
- the number of ignored tests increased significantly
- the build took significantly longer than the last ones
- the build times out
- ...
GO can't do any of those things.
Living community
Just try to google for some build related problems: Lots of answers for TeamCity, none for GO (which might be related to its name ;-) I wasn't able to find a GO community, again that may be the name, but visit the official
GO community site or count the
available community plugins. (most of the are written by ThoughtWorks employees anyway). TeamCity has a living community and in case googling doesn't solve your problem, Jetbrains has a fast and helpful support.
Native AWS support
TeamCity can operate a fleet of agents on Amazon EC2 instances. Depending on the current load (pending jobs) it can create, start or stop agents. This allows you to use very fast C4 instance types, which will make your builds faster, but in a very cost effective way, because those instances will live only for those few hours per day in which they are actually needed. GO again has nothing to offer in this area, you have to build scaling up and down by your own script or just pay 24/7 for agents you don't use.
Miscellaneous TC features where GO is inferior
- Auditing/Permissions: If you have more than one team TeamCity allows you manage permissions and it has a good auditing feature that shows you which user changed what and when. GO can show you the diff between two versions of the global config.xml file.
- Custom build numbers in TeamCity, incrementing only numbers in GO.
- Sophisticated environment variable setting in TC, predefined ones in GO
- Jobs can interact with Teamcity, e.g. update status data, nothing in GO
- Jobs can have arbitrary names and descriptions in TC, GO doesn't allow whitespace in names, leading_to_strange_conventions.
- all thinkable variants of triggers and dependency chains between jobs allows you to build sophisticated pipelines in TC
- job queues are visible in TC, making it easy to debug non starting and pending builds. In GO you have no hint when you job will start or to manipulate the queue when something went wrong. I have seen jobs hanging in GO forever because agents requirements were missing.
- Good history of deployments/builds from the past. You see which commits are in with deployment, and you can rollback to an older version directly from this history view.
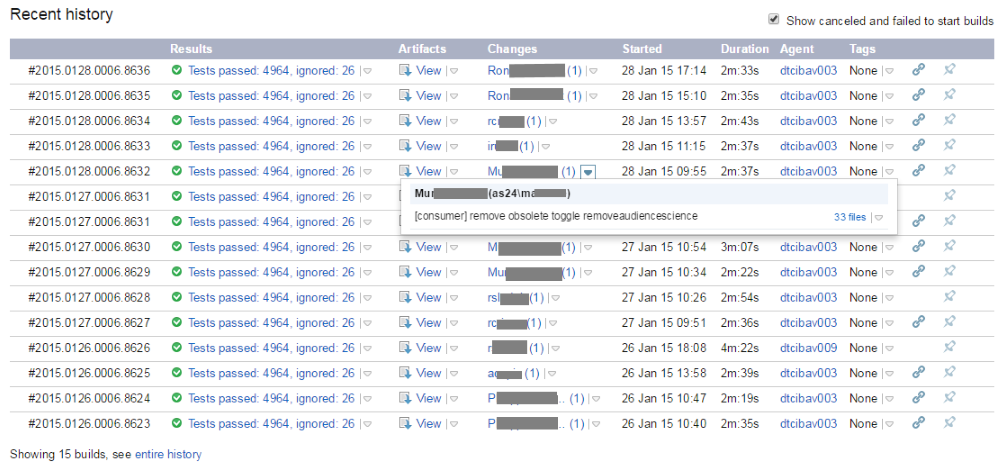
There are still more points where TeamCity outperforms GO (backups, recovery, auto updating agents, ...). I hope I have listed enough to show you mature TeamCity is compared against GO. If you have a problem there is a very high chance that TeamCity has a solution. With GO you will find yourself running into limitations or living with crude workarounds which make your teams less productive.
Conclusion
If you have a working and mature CI System (Jenkins, TeamCity, ...) don't switch to GO just because you want to do Continuous Delivery. GO has the same disadvantages but lacks many important productivity features. Either evolve your existing CI System to also deliver software or try out a radical different approach like
hubot deploy.